Engineering graduate students at Caltech are trying to make robotic prosthetics that walk more naturally.
Can you teach a robotic limb to walk naturally? That was the goal of a team of graduate engineering students from Caltech’s Advanced Mechanical Bipedal Experimental Robotics Lab (AMBER).
The Caltech student researchers combined a mathematical framework called hybrid zero dynamics (HZD) with a musculoskeletal model to control a robotic prosthetic, seeking not only to produce stable locomotion but also a more natural gait.
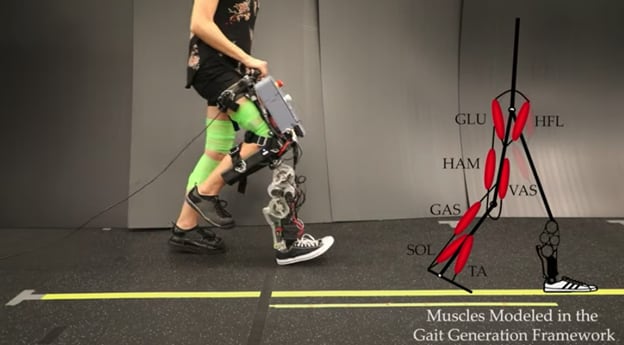
HZD is typically used to create walking gaits in bipedal robots. While this approach is ideal for ensuring the stability of walking gaits, it has optimization constraints and can often require multiple tunings to achieve a satisfactory gait.
“If you’re designing a trajectory for a robotic assistive device, a satisfactory gait should not only be stable but also feel natural,” said researcher Amy Li, a graduate student in computation and neural systems, in a Caltech press release.
The team wanted its robotic limb, a motorized prosthetic leg, to resemble as closely as possible the muscle activity of a natural human walk.
“The muscle activity pattern of a human walking without the prosthetic is what we want to get closer to,” says Rachel Gehlhar, a graduate student in mechanical and civil engineering and one of the members of the research team.
For this reason, the researchers embedded a musculoskeletal model into their optimization problem. This model represented how muscles stretch or contract with a given joint configuration, as measured through electromyography (EMG).
The musculoskeletal model imposed additional constraints on the robotic prosthetic that allowed it to develop a stable walking gait in less time than it would have with HZD alone.
Natural motion in prosthetics is something that typically relies on hand-tuning by domain experts, a demanding and time-consuming process. According to the researchers, there is still a poor understanding of how to translate biomechanics and natural motion to robotic platforms—particularly in robotic assistive devices. The framework proposed by the AMBER team could allow for much quicker and systematic tuning methods.
Research opportunities such as this create a broader educational experience for students, where they can test their problem-solving skills and explore new processes for designing, studying and testing solutions. Students are also able to use this experience to expand their knowledge and understanding of a field outside the classroom, often leading to significant breakthroughs. In addition, participating in research projects during their degree gives students the opportunity to work closely with a mentor, be it a faculty member or other experienced researchers. This helps students develop their career interests and connections while allowing them to attach their names to projects in the research community.
The team’s research was published in the April 2022 issue of IEEE Robotics and Automation Letters.