Generative AI and large language models help you pick your spots and add value to digital transformation.
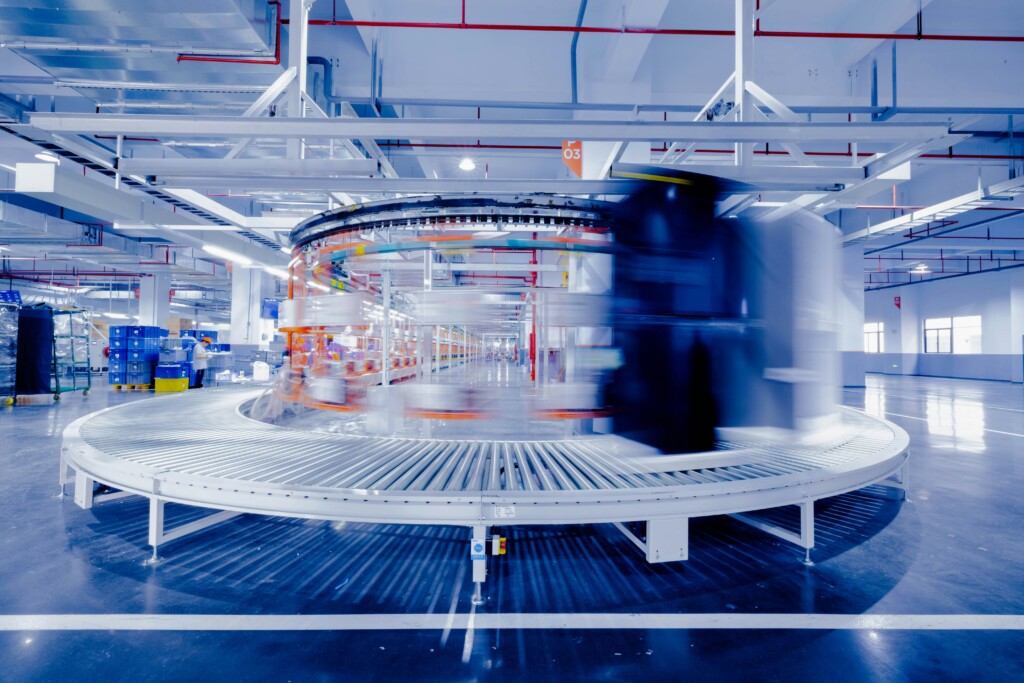
The power of artificial intelligence (AI) to enhance digital transformation initiatives has become increasingly evident to engineers as they seek to improve operational efficiencies, scale innovation and gain a competitive edge.
While digital transformation is hardly new, AI and large language models (LLMs) have emerged as a formidable accelerator by changing business processes, reshaping products and services and sometimes upending entire industries.
AI’s ability to learn and improve over time, coupled with digital transformation, means that organizations can realize faster processes, reduced costs and more efficient operations.
These AI benefits contribute to an environment of continuous improvement and innovation that is often key to success in a competitive environment.
Enhancing data-driven decision-making
Engineers know that data-driven organizations use digital insights to shape strategies, optimize processes and respond rapidly to market changes. However, harnessing the potential of integrated digital data at scale for decision-making requires far more than traditional data analytics.
AI’s capability adds unprecedented speed and precision to decision-making by:
- Sifting through structured data, identifying patterns and generating predictive insights.
- Analyzing vast volumes of unstructured data more effectively than search engines, specialized databases or software developers to generate predictive insights.
- Avoiding the cost and elapsed time associated with custom data integration of diverse data sources using software developers.
- Autonomously detecting trends and forecasting outcomes.
Adding AI and LLM capability to data-driven decision-making helps engineers optimize operational and strategic decisions while reducing the need to base decisions on history, experience, in-vogue ideas or hunches.
Examples of adding AI capability to data-driven decision-making for engineering include:
- Monitoring large volumes of IIoT data from production equipment to identify performance anomalies to avoid unscheduled downtime.
- Sifting through the external media for general and industry audiences to identify competitor initiatives that may require a response.
- Summarizing patent data, trademark data and research journals maintained in multiple languages to identify potentially relevant technology developments.
Automating processes and workflows
Automation is a fundamental aspect of digital transformation. AI-powered tools like robotic process automation (RPA), machine learning and cognitive computing, a type of AI that simulates human thought processes, have taken digital transformation to new heights.
While valuable, previous generations of automation that engineers implemented were limited to well-defined, repetitive tasks and detailed, rule-based decisions. AI expands automation to more complex decision-making processes, pattern recognition and more generalized problem-solving.
Examples of adding AI capability to automating processes and workflows include:
- Adding more accuracy and sophistication to simulations. For example, engineers can refine and enhance their designs through successive simulations to reduce limitations, which leads to more innovative solutions.
- Enhancing supply chain management for better product demand forecasting, logistics optimization, order fulfillment and risk assessments for component shortages. Achieving these improvements requires the integration of disparate data sources maintained by partners.
- Adding more intelligence to RPA transaction workflows such as invoice and shipment receipt processing. Examples include identifying potentially duplicate invoices, assessing the materiality of discrepancies and identifying likely fraud.
Improving data quality
Engineers are painfully aware that insufficient data quality is the number one reason for the failure of digital transformation initiatives. Asking data analysts to identify and correct data quality issues is slow, tedious, expensive and subject to further errors.
AI can automate data quality improvement work using pattern recognition. AI can achieve more speed and consistency at a lower cost than human analysts.
Examples of using AI capability to automate data quality improvement include:
- Recognizing that existing equipment can’t manufacture the designs due to dimensions, lack of accessibility and unachievable tolerances.
- Identifying and correcting instances where numeric values are associated with different units of measure or measurement systems creates errors and confusion.
- Sharply reducing the number of duplicate and incomplete inventory master records.
- Generating synthetic data to augment existing datasets to improve AI models.
Persisting knowledge
Organizations lose surprising amounts of essential knowledge and intellectual property (IP). Too often, engineers reinvestigate problems or wrestle again with design refinements because of a lack of awareness of prior work. Loss of knowledge and expertise typically occurs due to:
- Staff turnover and transfers.
- Reluctance to share knowledge.
- Lack of management support for knowledge management.
- Lack of time to document work.
- No repository in which to store work products.
- No easy ability to search and retrieve documents.
- Organization restructuring, acquisitions and mergers.
- Confusion caused by inaccurate, outdated or redundant versions of information.
Addressing these issues without digital transformation is impossible. Including digital knowledge management to the scope of digital transformation initiatives can significantly increase the value organizations achieve from the knowledge they have accumulated, often at considerable effort and cost.
Adding AI agents to knowledge repositories can add another increment of value. AI agents are intelligent software that use an LLM to perform query tasks, make decisions and learn from their experiences like humans. AI agents are a significant advance on the more familiar chatbots.
Examples of using AI agents to enhance digital knowledge management include enabling engineers to:
- Query “tribal knowledge” to improve production performance.
- Discover best practices.
- Better troubleshoot production equipment problems based on records of historical incidents.
- Query IP such as patent records, test results, research reports, development studies and licensing agreements in support of current work.
Challenges and considerations
While AI and LLMs add potential to digital transformation, engineers must acknowledge the challenges and ethical considerations associated with its deployment. These include:
- Ensuring data privacy to maintain customer and employee confidence.
- Addressing biases in AI algorithms and training data to maintain trust and inclusivity.
- Recognizing that LLMs may be incomplete or misleading.
- Training a skilled workforce that can effectively manage AI-driven processes.
- Fostering a culture that embraces innovation to ensure the smooth integration of AI and LLM technologies.
Engineers can establish robust data governance, prioritize transparency in communication and continuously monitor AI systems to mitigate unintended consequences.
Artificial intelligence is a powerful accelerator of digital transformation. Its impact spans most industries and functions, enhancing efficiency, agility and resilience. By embracing AI’s transformative potential, businesses can achieve a sustainable competitive advantage and drive long-term growth.