Consider these tips for digital twins.
Hexagon has sponsored this post.
Whether a production facility makes chemicals, energy or manufactured products, more and more of its production leads and engineers are hearing about operational digital twins. Digital twins are often discussed in the product development space as virtual doubles of real-world assets. They are created by integrating 3D modeling (or laser scans where 3D models are unavailable), simulation, machine learning, AI and the Internet of Things (IoT). Sensors on the real-world asset relay information to the twin and that data is processed to inform the user and designers of the object’s heath, status, operating conditions and more.
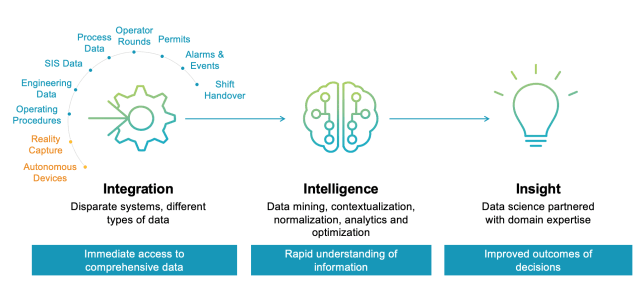
Operational digital twins work much the same way. Instead of monitoring final products, they monitor entire production plants to give operators rapid access to communication, data and information. Traditionally, production facilities struggle with data and documentation. Those that have replaced paper reports tend to use desperate digital tools (read: silos) that can duplicate or contradict each other. An operational digital twin makes information easy to find when it’s needed. It acts as a single source of truth for the facility. It also offers predictions, information and insights for system changes/decisions, what-if scenarios, root cause analysis, maintenance, risk mitigation, quality, efficiency, costs, cash flow, energy usage, resource/personnel management, hazardous conditions and more.
But creating a digital twin of a brownfield facility isn’t easy. There are many challenges for operators to consider as they digitize and digitalize processes and information. Here are three examples, and their solutions, that many production leads and engineers will face.
1. Integration and interoperability with legacy systems
“In most of our digital twin implementations, customers often operate facilities that have been functional for decades, with a significant amount of their information archived in unintelligent and unstructured formats,” said Adrian Park, vice president Pre-Sales EMIA at Hexagon in the e-book The Owner Operator’s definitive guide to better digital twins. “While digitizing brownfield sites is justifiably seen as more complex, the process of retrospectively constructing a digital twin from these available information sources is a common practice — and it can bring vast benefits.”
Park’s note on the challenges of digitizing brownfield assets is easier said than resolved. Decades-old equipment is unlikely to have the hardware needed to bring data to a digital twin. Even if equipment has tools to digitally track its status, it likely sends that data to siloed software.
For old equipment, adding sensors that can connect to the industrial IoT (IIoT) is a good first step. These sensors can track the health and status of the hardware digitally and then easily send the data to compatible digital twin software. To capture the geometry of these systems, laser scanning and geotags can be used to ensure the twin matches the real-world systems.
From a software perspective, operational digital twins don’t just integrate with equipment tracking software, they also speak to enterprise management tools. Examples of enterprise tools commonly integrated into digital twins include asset performance management (APM), product lifecycle management (PLM), enterprise resource planning (ERP), enterprise asset management (EAM) and manufacturing execution systems (MES).
The digital twin will also have to scrape data from files such as CAD, PDF and Word documents. This can also be a challenge. “We configure rules to automate the extraction of information from title blocks, reference tags and the building of hotspots for navigational ease,” said Park. “This unstructured data is then amalgamated with structured information, sourced from legacy data systems such as maintenance systems, thereby constructing a comprehensive digital twin retrospectively … Once the digital twin is set up, we recommend processes like electronic Management of Change (eMoC) to ensure that the digital twin is consistently updated and remains ‘evergreen.’” Park adds that new AI powered data extraction tools are planned for later this year. These will further simplify this process.
In other words, to solve this challenge, operators need to consider digital twin software that is flexible and can integrate well with third party systems. This way the twin can effectively consolidate information into a single source of truth. In the best-case scenario, these connections are done in partnership between the disparate software providers. This often results in software offerings that play nicely with each other. But in other cases, application programming interface (API) compatibility is necessary to ensure these connections.
2. Robust OT and ICS cybersecurity
Risk is a healthy concern when considering any digitization or digitalization process; operational digital twins are no different. A centralized collection of a production facility’s data, processes and overall ecosystem is a tempting target for any nefarious hacker. Similarly, this overarching system would cause significant delays if it were to fail due to non-malicious human or equipment error.
As a result, operational technology, industrial control systems and cybersecurity need to be a top priority when implementing an operational digital twin. To solve this challenge, consider paying close attention to:
- Inventory management to ensure everything is tracked and accounted for.
- Risk and vulnerability identification and remediation to proactively resolve issues.
- Configuration management to ensure security, best practices and attack vectors.
- Backup and recovery tools to ensure if/when anything happens salvage is swift.
When selecting digital twin software, be sure to also keep this list of considerations in mind.
3. Stakeholder management
Digital twins are more than visualization and analytics platforms, they manage information, documentation and provide users with a role-based view of all the information they need about the job they do. This means that the digital twin will cover large projects with numerous stakeholders both internal (like operators) and external (like suppliers and contractors).
As a result, it is critical to assign stakeholders with appropriate roles that are properly maintained and to ensure those users follow standard operating procedures. “The single greatest recipe for digital twin failure is a lack of commitment from key stakeholders,” said Waldir Pimentel, junior Customer Success manager at Hexagon.
Stakeholders that manually interact with and input data into the twin need to ensure their work is continual, consistent and correct. This guarantees that the digital twin accurately reflects the truth of the physical production plant.
To solve this challenge, be sure to consider digital twin software that offers smooth information handovers and avoids inundating stakeholders with too much data. This is especially true for external users that should remain unaware of certain intellectual properties. For internals and externals this requires a lot of training, so be sure the digital twin software is intuitive and easy to learn. However, more work may need to be done to ensure externals properly input data. For instance, proper data input and frequent audits can be added to contracts with third-party stakeholders.
Software and digital twin setup can also offer ways to ensure stakeholders input the proper information into the digital twin. When selecting digital twin software, be sure it can:
- Automate inputs wherever possible.
- Send manual data to a staging area that is validated before entering the digital twin.
- Guide user inputs via user interfaces, predefined rules and error warnings.
“To ensure data consistency from multiple sources, we run reports that cross-verify information,” said Park. “Further, these reports can check the presence of expected documents for specific tags, and identify potential missing documents.”
Perhaps the most important way to ensure stakeholders use the digital twin properly is to explain how it will benefit their personal workflows. For engineers, this might mean stressing that easy access to information can save them time and help projects move smoothly. For plant managers, this might mean having the right information and key performance indicators (KPIs) to ensure optimal production throughput. Once stakeholders see the benefits of digital twins for themselves, they are more likely to diligently use it.
To learn more about how to best implement and setup an operational digital twin, read the e-book: “The Owner Operator’s definitive guide to better digital twins.”