Here’s a few common steps manufacturers need to take to make AI a game-changing technology.
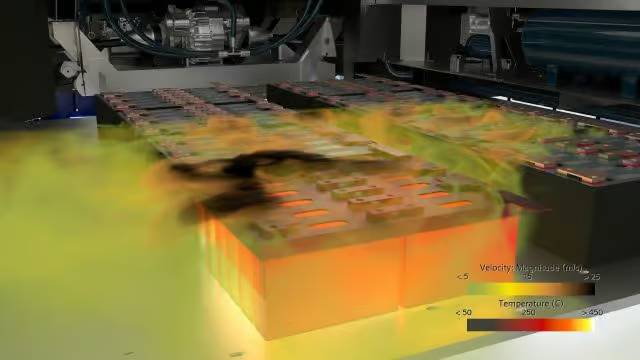
Artificial Intelligence (AI) is becoming an increasingly important tool for manufacturers who are seeking to unleash the potential of their operations and remain competitive. This Industrial Internet of Things (IIoT) technology enables operators to significantly boost the efficiency and lower the cost of their manufacturing processes.
But how exactly does a company incorporate these fascinating new tools into its operations?
Many manufacturers already collect and use data; the next logical step is to harness that data to help identify opportunities to improve the manufacturing process—and AI promises to be an integral technology in this process.
“The data strategy and how you collect data is very important,” said Remi Duquette, Vice-President of Industrial AI at Maya HTT, a company that provides computer-aided engineering solutions that include supporting manufacturers’ digital transformation and incorporation of IIoT technologies such as AI. “You need to define properly, as clearly as you can, what the problem is that you’re trying to solve.” Duquette spoke at a seminar on AI and machine learning hosted by NAFEMS in September 2023. NAFEMS is the international association for the engineering modelling, analysis and simulation community.
It’s crucial for a company implementing AI in its operations to make sure it’s collecting the right kinds of data—and that the data is reliable. Maya HTT works with companies to identify the variances in their clients’ datasets and help improve the quality—particularly in identifying and processing data outliers and determining if they are acceptable outliers or indicators of a larger problem. In fact, quality of data can be more important than quantity, especially early in the process: “you can always collect more data and improve the metrics and performance of your AI model,” said Duquette.
Transforming the shop floor into a digitized, AI-powered operation can be divided into a three-step process.
First is digitization. In this stage, the company needs to determine what sensors and digital networks it needs to gather the data for its AI solution. The company also needs to ensure the data it collects is clean and relevant to the task at hand.
In the second stage, advanced analytics, that data is put to work. The data is used to answer two important questions about the manufacturing operation: what is happening—a descriptive question—and why it is happening—a diagnostic question.
The third stage, machine learning, sees the AI platform building on the questions asked in the second stage. In this stage, the AI uses that data to predict what will happen, and prescribe solutions to control future events.
Duquette outlined three case studies to demonstrate how AI has improved a companies’ operations.
Aerospace
A small aerospace manufacturer had a low-volume job to produce composite domes that needed composite tape applied to them with resin—an operation performed by a robot. However, as the robot laid down layers of composite material, the tape would sometimes develop a twist in the fiber; removing the twist created slowdowns in production and undermined product quality.
For the first stage (digitization), the company already collected telemetry from the robot that included tip position, speed, resin temperature, resin flow rate and tape tension. The manufacturer then added a camera to provide visual data.
In the advanced analytics stage of the process, Maya HTT collected visual recordings and telemetry data, combining them to build a convolutional neural network that would inform the AI model. The visual data detected the twisting of the fiber itself, while telemetry data identified more subtle underlying issues involving resin temperature, flow rate and tape tension.
The third stage—machine learning—used the AI model detect process defects and pause the process for an inspector to examine and rectify the issue. Detecting and correcting the error before multiple layers of composite material were applied resulted in a significant reduction in rework, process downtime, additional pressures on inspection and quality control later in the process and quality of the final product.
Bringing AI into the process resulted in an improvement in detecting the defect by 29%. “That might seem low in terms of the defects that it found, but it reduced the rework by 82%,” said Duquette.
EV Battery Production
The second use case involved a discrete manufacturing process to produce lithium ion batteries for electric vehicles. The company’s main challenges were to reduce the product’s quality rejection rate and improve the variability in quality, which was significant from one battery to the next. An additional challenge was that the company performed its quality assurance process near the end of a manufacturing process that took about 24 hours to complete.
At the digitization stage, the manufacturer collected data from 16,000 real-time telemetry points throughout 10 stages of manufacturing. Data included weld temperature, speed of conveyors, oven temperature, battery temperature cooldown over time and electrical current discharge capabilities over time. A particular challenge was tracking the traceability of some wafer components which could be removed and reinserted in the process at different points.
In the advanced analytics stage, about 130 neural nets used the data, paying particular attention to the traceability issue to ensure that the data was linked to the right component.
For the final stage, the AI made predictions that identified defective components 99.2% of the time. It identified those flaws much earlier in the process, enabling the manufacturer to remove defective wafers after the fourth step in the process rather than at the end. The AI also identified a better way to track some of the data: rather than monitoring temperature cooldown and discharge rate as a function of time, it found that monitoring them as a function of temperature was more effective.
As a result, the manufacturer reduced its manufacturing time by 10 hours while retaining all of its quality assurance checks. These improvements resulted in a savings of raw lithium worth more than $400,000 annually.
Pulp and Paper
A pulp and paper company used AI to navigate the changes and challenges brought about by COVID-19. In particular, the company had to change its manufacturing process to meet a sudden spike in demand, while maintaining quality standards during a time of significant staff turnover and training pressures.
Maya HTT created a digital twin of the operation that included data from more than 600 sensors across a continuous manufacturing cycle to find efficiencies and improve finished product quality. One specific point of improvement was a reduction in discarded low-quality paper produced while machines were starting up.
An AI-based control system transmitted signals to the control room—not in real time, but rather as a guidance and assistance system to help the manufacturing process run more smoothly and efficiently.
Using AI resulted in process improvements that enabled the company to maintain its product quality while meeting increased demand, even as it was bringing in and training inexperienced new staff. In fact, productivity was increased and the work of human operators was augmented, allowing the continuous manufacturing cycle to be maintained.
As these use cases demonstrate, AI is a powerful tool that can help transform manufacturing processes for companies—regardless of how big they are or what industry they operate in. And manufacturers are catching on: according to Deloitte, 93% of companies surveyed believe AI could be a game changer in the world or industrial manufacturing. By putting in the groundwork to create a robust data strategy and clearly defining the problem that the AI would be used to solve, these companies gained a significant competitive edge.