6 experts weigh in on the future of AI-powered simulation tools.
With 2023 ending, one thing is clear: in 2024, AI will become an integral part of the engineering simulation industry. The topic has been discussed on engineering.com no less than seven times in the past year (Simscale’s AI announcement, a Design the Future episode, an interview with Neural Concept, Siemens’ AI announcement, Altair’s AI announcement, Ansys’ AI announcement, an interview with Ansys). And with announcements on this topic ramping up in the final quarter of 2023, engineers can expect 2024 to see many new AI-based simulation features.
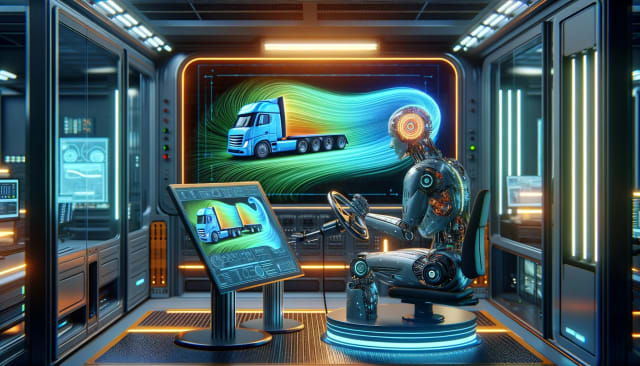
To get an idea of what to expect for AI-powered simulation in 2024 and beyond, engineering.com reached out to major CAE suppliers and thought-leading organizations. Six experts responded:
- Jesus Rodriguez, senior Manager of Research at Autodesk.
- Alex Tessier, director of Simulation Optimization & Systems Research at Autodesk.
- Fatma Kocer, vice president of Engineering Data Science at Altair.
- Justin Hodges, senior AI/ML technical specialist at Siemens Digital Industries Software.
- Ilya Tolchinsky, lead product manager at Ansys.
- Joe Walsh, lead of NAFEMS’ ASSESS Initiative.
The following is a summary of the results.
10 AI-powered Simulation Features to Pay Attention to in 2024
Though all the experts expect 2024 to be a boon year for CAE users, Kocer summed it up the best saying that engineering is entering into a fourth era with the convergence of simulation and AI. The tools and features the six experts think engineers need to pay attention to in the coming years will:
- Train bespoke AI-models based on existing simulation data.
- Speed up design space exploration using AI-based surrogate models.
- Bring simulation models to digital twins.
- Streamline/accelerate CAE workflows.
- Automate repetitive and tedious tasks.
- Pre-process known and similar model setups.
- Generate designs based on given data.
- Predict which simulations to run—and the needed fidelity.
- Select similar part geometry within a project or assembly.
- Group and codify parts based on functionality.
The experts agree that AI-powered simulations will help simplify the simulation process. For experienced users, it will reduce the number of tedious tasks they need to perform to get to the answers they want. For novices, it will democratize the tools so they can use them in the first place. Ease of use, setup, and computational times will all improve as AI becomes more integrated into the CAE experience.
“Using surrogate or AI-enhanced, physics-based models, for instance, we see engineers producing manufacturable, high-performing design options more quickly than they would without the help of cloud-powered AI,” writes Rodriguez. “We also see AI making simulation more accessible to less experienced users, with a net positive effect on productivity. If you think of AI as an interface, it can help handle the complexity of setting up and executing robust simulation, putting this powerful tool in the hands of more users.”
Walsh also notes that these simplifications and automations “should increase consistency and reduce the time necessary to obtain appropriate information on performance and behavior. We’ll be able to make more informed decisions on alternatives.”
Tessier agrees, but also offered some caution, writing: “AI will give engineers the opportunity to take advantage of accelerated and learned simulations to provide early feedback and guidance. However, AI is not a silver bullet; it does a lot to accelerate simulations, but engineers need to be cautious and diligent in performing their final analysis steps with proven, trusted and vetted techniques … with AI’s assistance providing strong and confident assessments, and even making simulations more accessible to non-analysts, the role of the analyst in the verification process will become even more important. This is especially true in these early days of AI-powered and AI-assisted simulation technologies.”
Though engineers need to keep in mind the limitations of AI in simulation, Tolchinsky highlights just how much of an impact it can make for engineers. He writes, the many AI applications in simulation “enable engineers to consider orders of magnitude more designs than existing workflows. Another vector is using natural language to interact with simulation software. This will make setup of simulations easier and expand the usage of simulation to non-experts. These are positive changes, but engineers will need to learn how to adapt to these new capabilities.”
But what about using AI to replace simulation solvers? Is this in the cards for 2024? Yes and no. Engineers are already using historical data to produce fast AI-models that act as surrogate models to a simulation solver. But—as is noted at the top of the features list—these are bespoke models. As a result, Hodges notes that, “For AI-powered simulations, as in AI-based solvers that replace our beloved FEA/CFD solvers, the impact will probably be limited in the near-term … But for AI-powered simulations, as in AI-based ROMs that allow us to explore more in a simulation project through adjacent design? [My] prediction [is] monumental.”
How to Organize, Prepare and Gather Data to Train AI-powered Simulation
For engineers looking to train their own AI algorithms to replace their common simulations, data governance will be an important factor. And since this has already begun in some engineering spaces in 2023, with examples expected to increase in 2024, now is the time to prepare this engineering data to make the most of it.
Walsh points out that the amount of training data required to train a model will be large but will also vary to reach the accuracy and fidelity needed to replace an engineering solver for a given situation. He wrote, “there is no one size fits all answer to this one.”
Tessier agreed, adding that larger organizations, with their larger data stores, will have the advantage here. “In the case of small to mid-sized businesses, it probably makes the most sense to find partners … and be willing to join a community to leverage and obtain enough data at the scale required to reap the benefits of ML/AI. This could mean rethinking how models are stored, shared and protected.”
Kocer explains what this data storage rethink might look like, writing, “Engineers need to have data discipline, meaning they need to not only save their simulation data but also organize the data and make it accessible to the entire enterprise.” As for how to generate the vast amount of data to train an AI model to replace a solver, she adds, “Engineers need to invest in design exploration methods such as Design of Experiments (DOE) for optimal synthetic data generation.”
Hodges gives a word of caution when generating this data, however. “The ‘garbage in, garbage out’ concept still holds here. Many data-driven approaches require analysis on the sampling approach used to generate simulation data, otherwise there is not strict confidence available to the practitioner on where they can apply the model. Further, traditional approaches apply for evaluating model accuracy once trained.”
Besides Data, How Do Engineers Prepare For AI-powered Simulation?
The consensus among the experts is that the best way for an engineer to prepare for the rising use of AI in simulation is to familiarize themselves with the technology. They need to understand its strengths and weaknesses. Or, as Kocer puts it, “engineers should start testing these technologies to grow with the trajectory of this emerging industry.”
Rodriguez dug deeper, hinting at one of the weaknesses AI engineers need to research. “Models are statistical in nature and are not based on first principles. Some simulations will work well with a neural network, while others may require auto-regressive models. The engineering community may resemble the finance community, with proprietary AI models developing for different applications.”
Since simulations are based on first principles and AI isn’t, Tessier suggests engineers “leave time for detailed/traditional analysis and verification. You can’t always ‘trust’ everything you get from AI models … ML is excellent at interpolation, but ‘rolls the dice’ for extrapolation. It is the engineer’s job to provide the final checks and not become overly trusting or get lazy with the final checks, validations and verifications when using these new technologies.”
Walsh was more specific, writing, “Every engineer needs to expand their understanding of verification, validation and uncertainty quantification (VVUQ) and understand when it is appropriate to use AI-powered simulation and when it is not.”
Are Fully Trained AI-based Simulation Solvers a Pipedream?
The idea of AI algorithms fully replacing simulation solvers is an enticing prospect. Simulations often take a long time to compute and use a lot of resources. AI, on the other hand, can reduce time to results with little computational power. But can AI fully replace Simulation models?
Tolchinsky thinks so. “This is an active area of research for Ansys. We are showing promising results, and I would say we are a couple of years away from realizing this capability.”
But not everyone was convinced. Rodriguez questions the practicability of training an AI model that can simulate “anything” as the data needed to train it would be enormous.
Instead, other experts suggested that AI can be used to replace some simulation models that are well understood and used locally—like they are being used now—but that there will still be a place for traditional solvers.
Hodges suggests similar approaches, writing, “I think some things are closer in reach, such as certain stages of the solver being replaced during a ‘solve event,’ certain empirical relationships being replaced for higher accuracy and lowering the resource intensive nature of certain parts via ML.”
Perhaps the most skeptical was Walsh, noting that, “Using AI to replace known numerical algorithms makes no sense at any time. The correct approach is ‘near real-time smart response surfaces’ based on a training set from detailed simulations. Some complex multi-physics scenarios exist where the numerical algorithms are currently unknown or unstable—AI may help here. Still, you need to understand the physics.”
Simulation a-la Star Trek: “Computer, run a simulation on…”
Many simulation engineers will remember Geordi La Forge asking the Enterprise to run a simulation. He only asked the question, and a complex model would appear. With the release of ChatGPT, language learning models (LLM) and the above examples of AI-driven simulation, it makes one wonder if this will become reality. There is some debate among the specialists, but the consensus is, if the model is already set up and ready to go, we aren’t that far off.
“LLMs could serve as the ultimate UT, so this is probably closer than we think,” argued Rodriguez.
Kocer was more specific, saying, “For a ready-to-go model, I think we are not too far from this. Given an engine bracket design, we are not too far away from saying ‘[computer], run a durability analysis [using FEA analysis].’” But she again stressed that this would be for a ready-to-go model. In other words, the language model should be able to select the right geometry from a library, but “generating that model using language models, I think is pretty far as we don’t know how to verbally describe the details of large, detailed 3D geometries.”
To make this Star Trek concept a reality, Hodges explained that application programming interfaces (APIs) would need to be set up between a tool like ChatGPT and simulation software. He notes that thousands of people are likely working on this already in the CAE industry, and he is hopeful it will be coming soon.
Walsh, again, was more skeptical. He said, “I’d love AI to beam us up, but there are too many outstanding questions to hazard a guess on this one. ASSESS is all about facilitating a rapid increase in the availability and utility of engineering simulation. We’ll get to the Starship Enterprise eventually, but the captain’s log tells me that’s some way off!”