From predictive maintenance to better quality control, AI is changing how—and where—things are made.
Manufacturers are betting big on artificial intelligence (AI), according to a new survey from Augury, an Israel-based company that uses AI to improve manufacturing processes. Augury’s The State of Production Health 2023 report revealed that 63% of manufacturing respondents plan to increase AI spending this year.
That manufacturers are eager to take advantage of AI is no surprise, given the technology’s recent boom. AI is already on the production line, helping manufacturers improve efficiency and reduce costs in tasks ranging from measuring adherence to safety guidelines to reducing scrap. There’s a high ceiling on how much value AI could ultimately bring to manufacturers, but almost every company can get in on the ground floor.
How AI can prevent production issues
One of AI’s most useful capabilities is its speedy data analysis. With access to production data from sensors and context from part numbers and employee input, AI can spot issues when it matters most.
“The worst and most expensive time to find issues is during production,” says Anna-Katrina Shedletsky, co-founder and CEO of Instrumental, a Palo Alto-based company that uses AI to spot manufacturing defects. She told engineering.com that the best time to find is issues is before they occur.
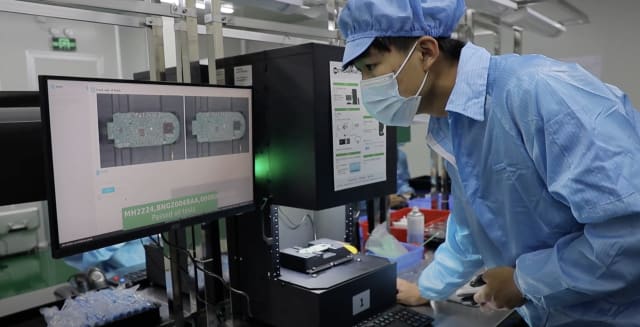
For example, imagine a robot in an automotive factory that attaches door handles. By taking in sensor data such as how much pressure the robot is applying, and factoring in context such as how many handles the robot has installed since its last maintenance check, a well-trained machine learning (ML) model could estimate when and how the robot is likely to fail. This would give the manufacturer the opportunity to perform maintenance before—not after—failure occurs.
With AI-enabled predictive maintenance, manufacturers can identify what problems their equipment will experience, when these problems may occur, and what issues would result. All of this information allows facility managers to avoid disaster. They can schedule repair, maintenance or replacement and downtime at optimal points. This means they can avoid downtime, equipment breakage, substandard products, and unnecessary energy and labor costs, as well as potential injuries to workers.
Determining where AI is needed
So how can AI improve your production line? It depends on your organizational goals. Whether you want to facilitate predictive maintenance, improve quality control or accelerate production time, there’s likely a way AI can help.
Shedletsky says that most manufacturers currently lack the AI tools to improve their processes, especially when it comes to assembly. If you’re not already equipped with the technology, she says one of the easiest ways to get started is to partner with an AI consultant.
After you determine what you goals you want to achieve, it’s crucial to talk with your engineering and operations department staff. They can share additional information about what is risky or special about a given product. For example, a waterproof smart speaker would need to be tested for exposure to and immersion in water.
The next step is to determine what training data would be needed for an AI model. This can involve taking photos of your product at key states of assembly. After that, you should collect and utilize data to measure how well the product functions.
This is where AI can begin to shine. When AI spots an issue like substandard device performance, it can track which units exhibit the problem and the range of variance. AI may also offer clues about the cause of the problem, such as a defective assembly robot. This will allow you to take corrective action like repairing affected units.
As an example, one of Instrumental’s recent customers was a manufacturer of first responder radios like those used by firefighters and EMTs every day. The manufacturer sought to improve their best practices to maintain quality and reduce the cost of inspection. They also wanted to prevent “escapes,” the shipment of products that did not meet specifications.
The manufacturer used AI to set up tests for known defect types. The AI also helped identify and intercept novel defects automatically. The manufacturer prevented escapes by intercepting suspicious units on the line for an inspection operator to review. The combination of AI and one human inspector did a better job at identifying defects than a series of four human inspectors all doing the same end-of-line quality checks.
The limitations of AI
AI isn’t perfect, and it still needs to be paired with human oversight. Shedletsky gave the example of another manufacturer that received the incorrect components from a sub-supplier, which were assembled into half of the products built across several days. The manufacturer was using AI to spot anomalies, but a 50% issue is not an anomaly.
“It’s just part of the population, so the algorithms did not automatically discover the defect. The customer identified the incorrect component through other testing,” said Shedletsky.
But where AI failed, AI also came to the rescue. The manufacturer used Instrumental’s AI to do a deep visual search of all the manufactured units. Within seconds, it created a list of all units with the incorrect part. This cut the size of the recall and rework list in half, saving the customer a significant amount of time and money.
How AI is impacting electronics manufacturing
The amount of press that AI tools like ChatGPT has received has increased manufacturers’ interest in such technologies. Becoming aware of AI’s potential and utilizing AI more allows engineers to eliminate certain boring, repetitive parts of their work. These are the tasks on which they and other workers are most likely to make mistakes.
AI also happens to be capturing engineers’ attention when the electronics manufacturing industry is undergoing enormous shifts.
“Over the past few years, we’ve seen medium-size electronics companies pull out of China. They’re relocating to Malaysia, Vietnam, Thailand, Taiwan, Mexico, and even the U.S. The U.S. is seeing a scale-up in manufacturing due to tax incentives offered by the Biden administration,” says Shedletsky. Biden signed the CHIPS Act in 2022 to revitalize the U.S. semiconductor industry.
During this shift, engineers and operations teams can reduce risk by ensuring set-up and assembly in the new factories goes well. This requires building rapport with new manufacturing partner teams. Engineers and operations teams must also learn how to solve problems with new partners effectively. AI has a place in finding and fixing concerns on the factory floor.
Despite AI’s seemingly endless potential, there is a cost to contract for AI services or develop AI technologies in-house. Manufacturers should track what improvements AI has made to understand how much these pay off over time. It’s also important to keep current with the development of AI to understand what it can and cannot do. For now, it’s still no substitute for human engineering judgement.