DeepMind's neural network, AlphaFold, predicts the structures of 350,000 proteins in humans and beyond.
The central dogma of genetics describes all life on Earth. These biological principles follow a one-way flow of information from DNA to RNA to protein. Solving the code underlying these transitions has been critical to advances in our knowledge of everything from fundamental biology to human health and disease. These biological information systems are composed of different materials—DNA and RNA are composed of nucleotides, whereas proteins are composed of amino acids. Unfortunately, determining a protein’s shape from its amino acid sequence remains largely enigmatic despite decades of extensive structural biology work.
Recently, Google’s deep-learning program, DeepMind, published two papers in Nature that describe their transformational neural network, AlphaFold. The first paper describes their neural network and the underlying logic of the predictive program, while the second dives into the accuracy of their predictions that span the human proteome. Collectively, the two publications describe how AlphaFold can accurately predict protein structures from their linear amino acid sequences. This development stands to transform modern research and our understanding of biology.
“This will change medicine. It will change research. It will change bioengineering. It will change everything,” said Andrei Lupas, an evolutionary biologist at the Max Planck Institute for Developmental Biology in Tübingen, Germany. He was responsible for evaluating the performance of over 100 different teams at the biennial Critical Assessment of Structure Prediction (CASP), a protein-structure prediction challenge. In November 2020, AlphaFold outcompeted all teams in the competition and sparked excitement across diverse areas of biology.
The two recent publications and the newly published AlphaFold Protein Structure Database provide a comprehensive resource for the field of biology across academia and industry.
Protein Structures Dictate Their Biological Function
Proteins, the building blocks of life, adopt a 3D shape based on their amino acid sequence and the laws of physics. Unfortunately, predicting these 3D shapes based on linear sequences of amino acids has remained largely elusive to structural bioinformaticians. Cyrus Levinthal famously described the paradox of protein structure prediction in 1969, when he noted that it would take longer than the age of the known universe to evaluate all possible configurations of an average protein. However, in the natural world, proteins fold spontaneously, alluding to a set of principles that should dictate a protein’s structure based on its sequence.
This code has yet to be cracked, resulting in scientists performing tedious laboratory experiments to identify individual protein structures. Usually, this involves either x-ray crystallography or cryo-electron microscopy to image a protein and determine its shape. These experiments can take a long time and are often difficult or impossible if a protein is membrane-bound or intrinsically unstable. Despite the success of the Human Genome Project and other advances in genomics, structural biology remains largely underexplored. Only one-third of the 20,000 proteins in the human genome have an experimentally determined structure, and most of those structures are not complete.
But why do we care about a protein’s structure if we know its sequence? An important adage in science is that ‘structure is function.’ If a protein is unstable or does not fold correctly, it needs to be identified and removed from a cell before it can cause damage. Many human diseases are caused by or associated with misfolded proteins, including Alzheimer’s Disease, cystic fibrosis and Huntington’s disease.
Beyond causing pathology, understanding a protein’s structure has broad applications for drug discovery, as inhibiting a protein’s function can be critical to treating disease. With detailed structural information, scientists can develop potent inhibitors of a given protein using structure-guided drug design to create better therapeutics.
To enable our understanding of protein function and accelerate research, scientists need to know how to use an amino acid sequence to predict a protein’s final structure. AlphaFold and their ever-expanding database of predicted structures may be the solution biologists have eagerly awaited.
CASP14 Highlights Growing Investment in AI for Structural Bioinformatics
Since the 1980s, researchers have been interested in using computers to predict protein structures and facilitate biological studies. Since 1994, the CASP competition has challenged teams to computationally predict the structure of proteins that researchers already solved using experimental approaches.
In 2018, DeepMind competed with the first iteration of AlphaFold, a highly accurate program that used similar AI models to those submitted by other teams. The first version of AlphaFold used structural and genetic data to inform a deep learning algorithm that predicted the distance between amino acids in a protein’s structure. A second step in the program that did not rely on AI then created a ‘consensus’ model that predicted the protein’s shape.
However, the team was unable to improve the accuracy of this model and switched gears for the 2020 competition. The new and highly accurate version of AlphaFold (v2.0) incorporates information about the physical constraints affecting protein shape to predict the final protein structure.
With their new version of AlphaFold, DeepMind shocked the field with startling accuracy at CASP14. Two-thirds of their predictions were similar in quality to experimentally derived structures. Evaluators of the competition were also unsure if the remaining one-third of predictions varied from published data due to experimental artifacts or the accuracy of the predictive software.
DeepMind has also made the source code available for AlphaFold to allow researchers to use the software to predict their proteins of interest.
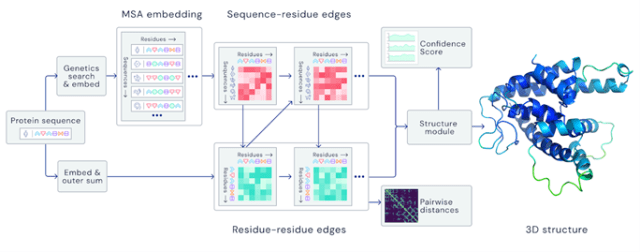
“We have been stuck on this one problem—how do proteins fold up —for nearly 50 years. To see DeepMind produce a solution for this, having worked personally on this problem for so long and after so many stops and starts, wondering if we’d ever get there, is a very special moment,” said John Moult, co-founder and chair of CASP, and professor at the University of Maryland.
Beyond AlphaFold, many companies and researchers are also working on developing computational approaches to predict protein structure. A group of researchers recently published their system, RoseTTAFold, in Science that approaches the accuracy of AlphaFold for predicting protein 3D shapes. As a growing area of research, scientists and engineers are utilizing the power of AI to solve significant problems in biology in real-time.
What Makes AlphaFold Unique?
Previously, researchers used two distinct approaches to tackle protein structure prediction. The first relied on physical interactions between amino acids and the principles of thermodynamics. Limitations to this approach included a lack of context for factors that can affect final protein structures in vivo combined with the cumbersome and time-consuming nature of molecular simulations. The second approach relied on the evolution of protein structures and used information on protein homology across evolutionary time scales to predict conformations. However, the number and quality of available experimental protein structures limited this approach.
AlphaFold differs from previous approaches to protein structure prediction by integrating evolutionary, physical and geometric constraint data that can all impact 3D conformations. Using a novel neural network called ‘Evoformer,’ AlphaFold uses pairwise features and multiple sequence alignments (MSAs) to enable end-to-end prediction of protein structures from a linear amino acid sequence.
The program focuses on using iterative refinements to recreate the final output and consistently improve the overall accuracy of the prediction. For example, one fascinating area of innovation in the program is its ability to break the predicted chain to allow for simultaneous local refinement at an atomic level. Despite violating peptide bond geometry, this process allows for a faster, more accurate prediction process.
AlphaFold Accurately Predicts Protein Structures from Linear Sequences
Currently, the AlphaFold database includes structures for 350,000 proteins from humans, mice, corn and other vital species. The company anticipates the database will grow to include upwards of 130 million structures by the end of the year. The current database covers 98.5 percent of known human proteins, with 36 percent of those predictions considered precise enough to enable structure-guided drug design.
“This is the biggest contribution an AI system has made so far to advancing scientific knowledge. I don’t think it’s a stretch to say that,” said Demis Hassabis, co-founder and CEO of DeepMind.
AlphaFold could even provide structure predictions for SARS-CoV-2 proteins in early 2020, well before experimental data was available. One of these predictions closely matched experimental data later released for one of the viral proteins.
All structures are currently available through the AlphaFold Protein Structure Database maintained by the European Molecular Biology Laboratory-European Bioinformatics Institute. A rich resource for the field, structural and computational biologists can now use these structures to enable diverse research efforts, including large-scale analysis of protein evolution.
AlphaFold’s Structural Insights Suggest Novel Biological Functions
In their paper, the DeepMind team highlighted exciting results obtained from the initial 350,000 protein structure dataset. AlphaFold predicted several protein structures that were previously unable to be solved and now provide hypotheses to spark further research.
For example, glucose-6-phosphatase is a membrane-bound protein that catalyzes the final step in the gluconeogenesis pathway. This pathway synthesizes glucose in the liver and is essential for maintaining blood sugar levels and enabling the survival of diverse biological organisms. The AlphaFold prediction identified a conserved residue in the enzyme binding pocket that was not previously described and may be critical for the protein’s function.
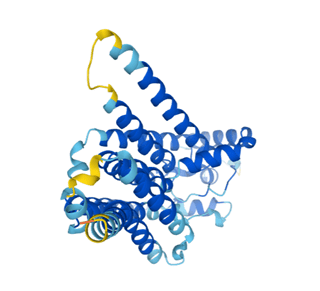
Predictions like this can enable scientists and physicians to develop hypothesis-driven approaches for disease treatment and drug design. With widespread applications in medicine, the predicted proteins in the human genome are only the beginning of AlphaFold’s potential.
What’s Next for Structural Bioinformatics?
Although the accuracy of AlphaFold may spawn fear for the future of structural biology, most researchers are not concerned. Many structural biologists spend years trying to solve the structure of a protein in order to begin understanding its function and role in a given organism. With AlphaFold, scientists can use predicted structures to enable further research and accelerate our fundamental knowledge of organisms with diverse applications. After all, most structural biologists do not consider themselves in the business of solving structures but in interpreting them.
With the goal of releasing 130 million predicted structures by the end of the year, scientists will be able to access detailed structural information for nearly half of all known proteins. A transformative advancement in biology, we will likely see the benefits of this AI-enabled work over the next few decades as all areas of biology benefit from this resource.